Co-author: Christian Andresen –
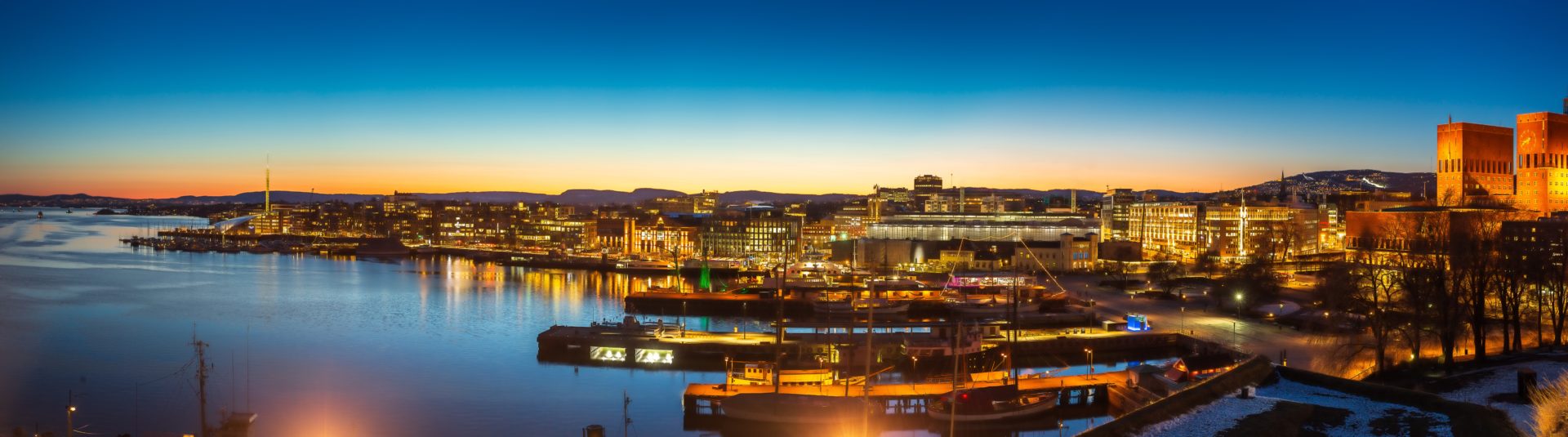
The EarlyWarn project aims to predict faults in the power system by developing models that use big data, machine learning and domain knowledge to analyse historical power quality data.
So far, the model’s predictive ability has been solely based on analysing power quality data from the period before a fault occurs. While the results have indicated that it is possible to predict faults, predictions based on a single data source are not yet accurate enough to be implemented in an operative environment. However, it is likely that the model’s predictive ability can be improved by using multiple types of data sources to form a better prediction basis.
Measures for reducing downtime in the power system
Norway has one of the most reliable power systems in the world, with a continuity of power supply of 99.988% in 2019. Norway is also one of the most electricity-dependant countries in the world, with a high electrification rate. This is partly due to heating in the majority of Norwegian households being electricity based.
Norway’s transition to a low-emissions society, in parallel with increased digitalisation and automation, is expected to increase electrification and make a safe and reliable power supply even more crucial in terms of both value creation and daily life.
Furthermore, if there is a power outage in Norway, Norwegian grid companies are required to cover the cost of energy not supplied (CENS), which is the calculated value of the lost load. Due to this, grid companies have increased their focus on continuity of supply and are now investigating new ways of reducing downtime in the power system.
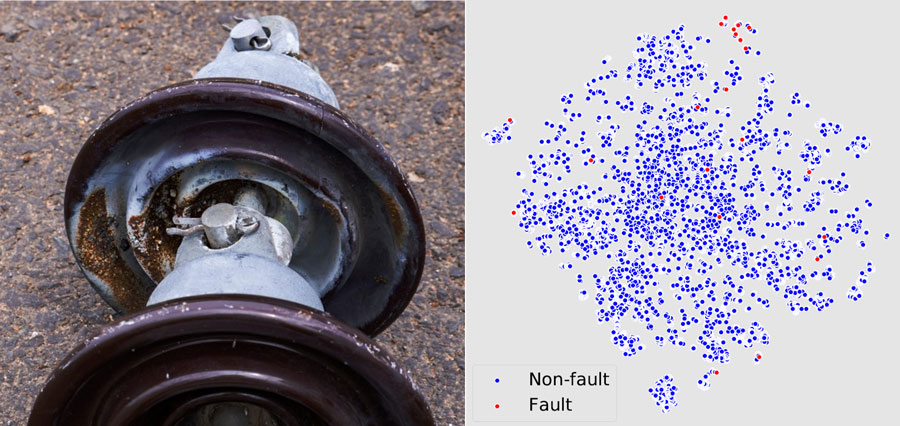
Power quality analysers have been gathering big data for over 15 years
In order to increase our knowledge of the grid conditions and causes of faults in the power system, many power companies have installed a number of power quality analysers in their concessionary areas.
Power quality analysers are measuring devices that can log the voltage and current waveforms to a high degree of accuracy. As they record up to tens of thousands of samples per second, these devices are also able to register small disturbances in the system. These disturbances can be caused by equipment that is damaged or weakened but not yet failed completely, or by outside influences that gradually build up over time, such as vegetation, salt coatings or snow loads.
Some of these power quality analysers have been continuously registering the quality of supply of the Norwegian power system for over 15 years.
The figure below shows a time series of voltage measurements from a high-voltage grid over a ten-year period. During normal operations, the voltage should be about 22 kW. This means that any deviations from 22 kW (over- or undervoltage) shown in the figure are due to either faults or disturbances in the power system.
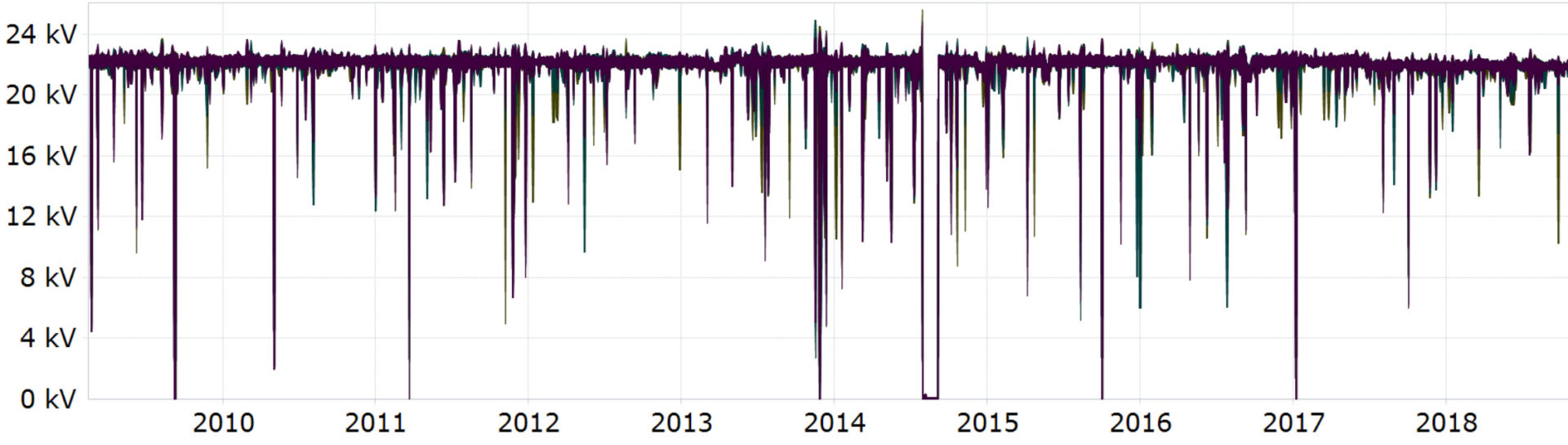
Combining knowledge about fault signatures and their underlying causes with new data-driven methods, such as machine learning, could enable us to predict problems in the power system before the event actually occurs . This in turn can allow network operators to take preventative measures in order to prevent power outages and other problems for the consumers. Not only will this increase the robustness of the system and reduce operational and maintenance costs, but it also maintains the security of supply, limits damage to equipment, and avoids situations that are potentially dangerous to people.
The EarlyWarn project aims to predict faults in the power system
EarlyWarn is a research project that aims to develop a model to predict and detect disturbances in the power system before they can escalate and cause faults, such as power outages.
To achieve this, the project exploits the large amounts of data that has been gathered by the power system. By using big data, machine learning and domain knowledge, EarlyWarn has developed methods for automatically and continuously analysing this data, which can then be used to alert system operators of any instabilities or operational disturbances that otherwise would have gone unnoticed.
More information about the EarlyWarn project can be found here.
Developing predictive algorithms
Developing and training machine learning models requires extremely large datasets. It is essential that this data includes time series from both normal operations and fault events in order to train the algorithm to both differentiate between the two and recognise conditions that can lead to faults occurring. Therefore, the EarlyWarn project has developed methods for generating user-customised datasets based on observations from power quality analysers.
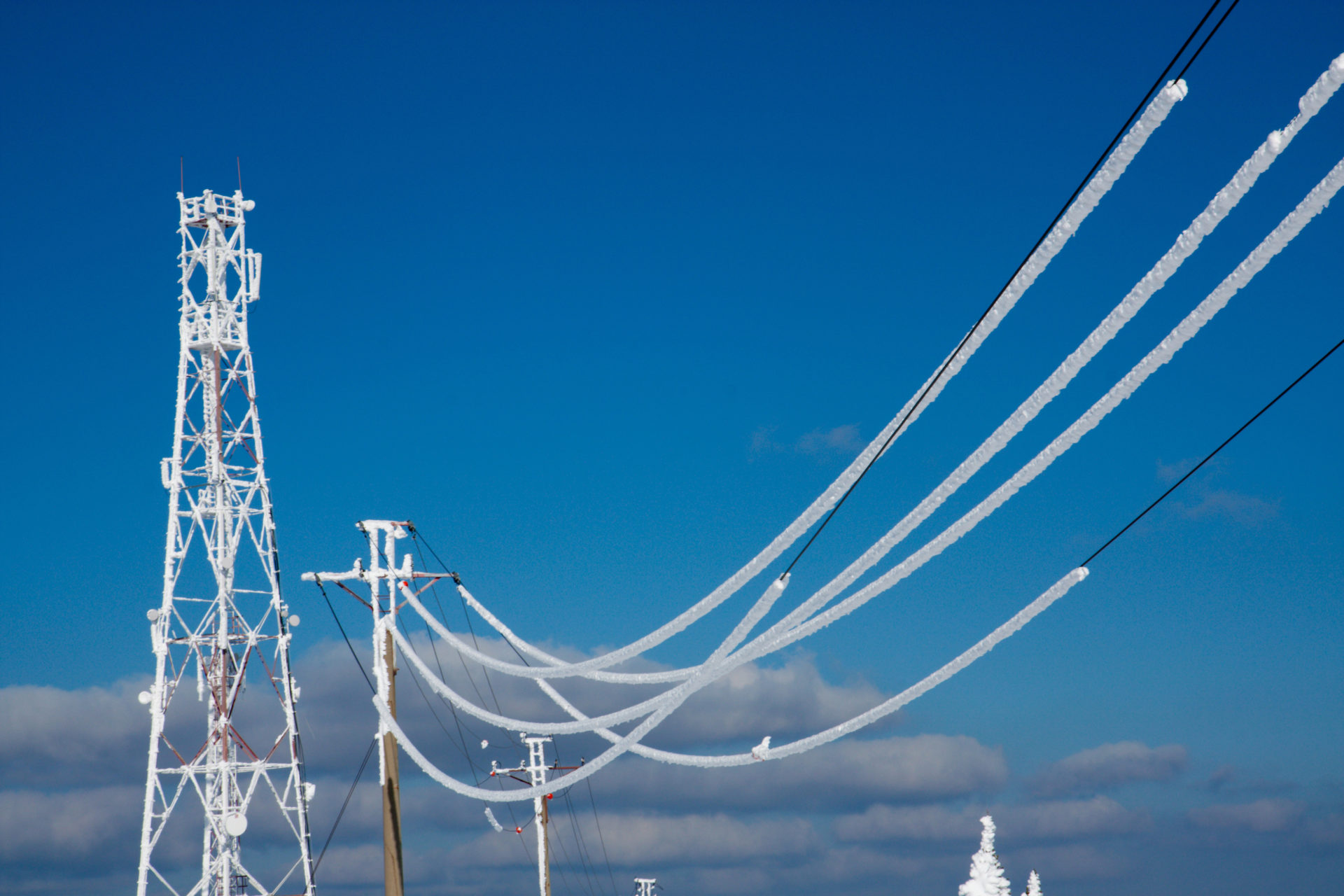
The details of the methods that have been used and their subsequent results can be found om the articles cited below in the project’s publication list. We will update this list as more articles are approved:
- Andresen, C. A., Torsæter, B. N., Haugdal, H. & Uhlen, K. (2018). “Fault Detection and Prediction in Smart Grids”, 9th International Workshop on Applied Measurements for Power Systems (AMPS). Bologna, Italy.
- Hoffmann, V., Michalowska, K., Andresen, C. A. & Torsæter, B. N. (2019). “Incipient Fault Prediction in Power Quality Monitoring”, CIRED 2019 Conference. Madrid, Spain.
- Tyvold, S., Torsæter, B. N., Andresen, C. A. & Hoffman, V. (2020). “Impact of the Temporal Distribution of Faults on Prediction of Voltage Anomalies in the Power Grid”, 2020 International Conference on Smart Energy Systems and Technologies (SEST). Istanbul, Turkey.
- Høiem, K. W., Santi, V., Torsæter, B. N., Langseth, H., Andresen, C. A. & Rosenlund, G. H. (2020). “Comparative Study of Event Prediction in Power Grids using Supervised Machine Learning Methods”, 2020 International Conference on Smart Energy Systems and Technologies (SEST). Istanbul, Turkey.
- Rosenlund, G.H., Høiem, K. W., Torsæter, B. N. & Andresen, C. A. (2020.) “Clustering and Dimensionality-reduction Techniques Applied on Power Quality Measurement Data”, 2020 International Conference on Smart Energy Systems and Technologies (SEST). Istanbul, Turkey.
- Michalowska, K., Hoffman, V. & Andresen, C. (2020). “Impact of seasonal weather on forecasting of power quality disturbances in distribution grids”, 2020 International Conference on Smart Energy Systems and Technologies (SEST). Istanbul, Turkey.
Two types of events in a fault cluster
Results from the EarlyWarn project have shown that it is possible to predict faults in the power system to a certain degree, based exclusively on developments in the power quality data before the fault occurs. However, the accuracy of this model is still too low for operational implementation.
This low accuracy could partly be attributed to the fact that faults in the power system tend to occur in clusters, with the first fault often triggering multiple consecutive events. It is therefore important that the model be able to differentiate between the prediction of “first-in-sequence” events (events that do not occur immediately after another event) and “domino effect” events, which occur after an initial failure.
This has proved challenging when using power quality data as the sole basis for prediction. When examining the prediction results for all types of events, the algorithm could predict 2/3 of all events with a reasonable error rate. However, this accuracy depends significantly on when the event occurs in a cluster. If we focus on the “first-in-sequence” events alone, the prediction ability drops to 5% – 10%, depending on the nature of the event. Therefore, in an operational situation, the value of the prediction differs between the two types of events. In particular, we need to focus on increasing the accuracy of predicting “first-in-sequence” events.
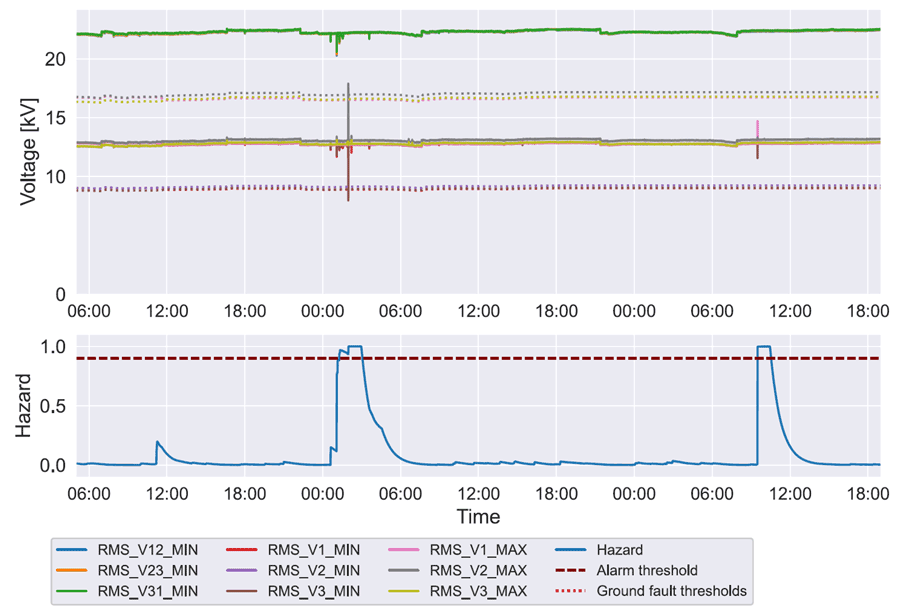
Promising results – but more data sources are needed
It is clear that a predictive model based solely on the development of power quality data will be limited in accuracy and may struggle to predict all types of faults without risking false alarms. However, the results are promising and indicate that work should be continued with developing and testing various machine learning methods, with the goal of increasing the model’s prediction ability and prediction horizon.
It is likely that the combined use of various types of independent data sources in the monitoring system will improve the accuracy and prediction ability of the model. Below is an extract from a study that investigated whether various weather phenomena could be used as predictors of certain types of faults. The results show that while weather data does have a predictive ability, combining weather data with power quality data (and other data sources) will be able to produce significant synergies.
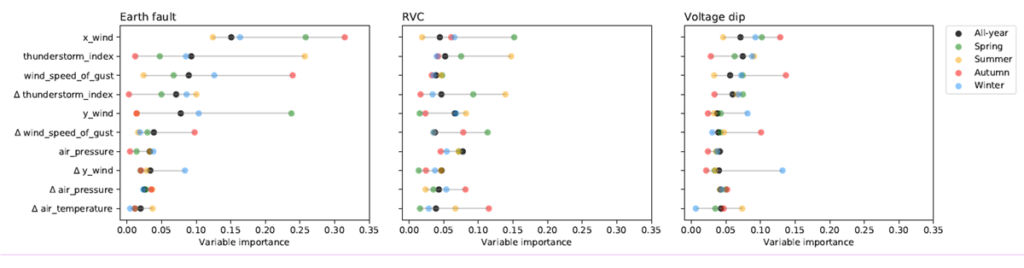
Alternative data sources could include, but are not limited to, weather data, power grid topology, sensors on critical components, and load data. This is an important topic in the completion phase of the EarlyWarn project, and also a subject that is worthy of further research.
This article was originally published on February 12th, 2019, and updated on April 14th, 2021.
0 comments on “Machine learning can predict faults in the Norwegian power system”