Hydropower is facing new challenges – competition in the market to provide clean energy as part of Norway’s “green transition”, and the wave of digitalisation that will bring about changes to our working lives. Machine learning, cyber-physical systems, the Internet of Things, data mining and the Internet of Services are just some of the key terms linked to digitalisation.
Challenging? Yes.
Exciting? Absolutely!
I’m working with my colleagues on a project looking into how the digitalisation of hydropower can be used to improve power plant maintenance. The project is called MonitorX.
The aim of the project is to assess current condition status and identify new opportunities for the hydropower sector in the new digital reality. It is a collaborative effort with Energi Norge, and is being funded by the Research Council of Norway.
- Read more about SINTEF’s risk-based asset management of power networks and hydropower plants.
Hydropower digitalisation opens the door to new opportunities for maintenace
Right now, the hydropower industry has a lot on its plate. Energy efficiency; renewable energy certificates; the subsidisation of new renewable energy sources to promote competition; lower gas and oil prices that influence electricity prices; changes in the market resulting from globalisation, not to mention environmental challenges. These are just some of the issues currently preoccupying the sector. On top of all this Norway is managing an ever-ageing number of power plants, all looking much as they did when they were built back in the 1960s and -70s.
These ageing plants can be expensive to run, but millions of kroner can be saved in maintenance costs if the industry starts focusing on better planning and targeted maintenance, aided by digitalisation.
Identify faults before breakdown occurs
During the first phase of the MonitorX project, we talked to representatives from the industry to ask questions such as:
- what data do you collect, and what data collecting systems do you use?
- how do you store data, and for how long?
- do you use data analytically, in particular in connection with maintenance issues?
We received many responses, and some of the results were presented at the “Production Technical Conference” (PTK). (Link in Norwegian)
Next phase – is it working?
We have now started the next phase, which involves the development and testing of a variety of algorithms and methods designed to analyse operational data from power plant control systems. In other words, we will be using available data to identify faults at an early stage as a basis for improving maintenance processes.
We’re talking about models and algorithms that will use the aforementioned operational data from control systems, combined with any data from other sensors and measuring equipment installed in the plants. These will provide us with notifications of changes in function or performance.
The models and algorithms will help the companies to identify faults or, more precisely, future faults in the plants.
The aim is to identify such faults before a serious malfunction occurs. It will be like your car saying to you “I don’t feel well, drive me to a garage right now before I break down completely”.
To see if this really works, we’re running some test case studies as part of the project. To date, we have identified just over ten cases for study, and some of these have already started.
Machine learning identifies patterens and deviations in the data sets
We’ll be using the case studies to focus for the most part on advanced models, such as the use of machine learning to identify patterens and deviations in the data sets. But we’re not excluding other types of models such as Fyzzy Logic, physical models or a combination of algorithms.
We’re also looking at the potential for the more extensive use of new types of sensors, such as audio sensors and ultrasound, as data sources.
Getting our priorities right – avoiding breakdown and unnecessary replacement
The figure below illustrates how different concepts such as cyber-physical systems (CPS), the Internet of Things (IoT), data mining (DM) and the Internet of Services (IoS) can be placed into a predictive maintenance framework that utilises measured data.
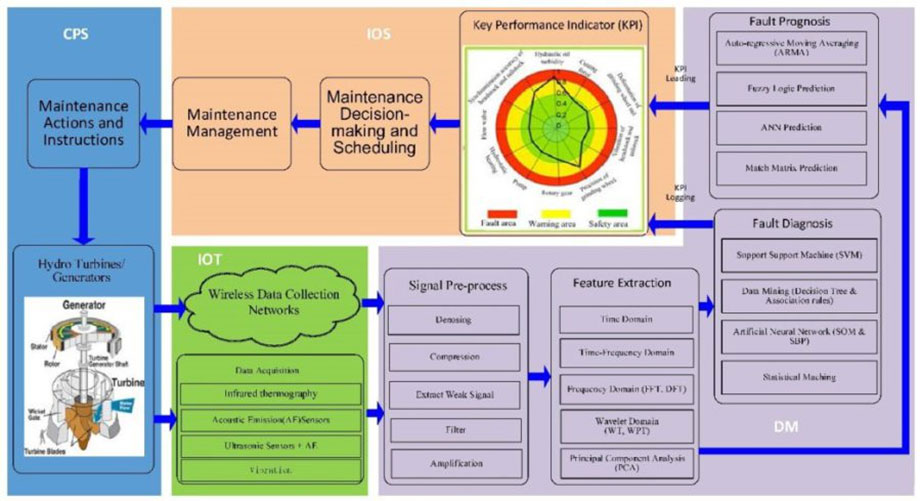
By utilising digitalisation and the correct concepts, the hydropower industry will be able to rationalise its maintenance processes and cut costs. And millions will be saved in maintenance costs!
You can follow developments in the project on our website.
0 comments on “Digitalisation can save millions in hydropower maintenance costs”