Machine learning has rapidly become a popular research topic in science and engineering due to its vast potential for solving complex problems and improving processes. The number of research projects and publications related to this field is growing rapidly as more researchers recognize its potential benefits. Machine learning has also found applications in geotechnics and engineering geology, with researchers using it in various ways to improve processes and solve complex problems, such as predicting the behaviour of soils and rocks.
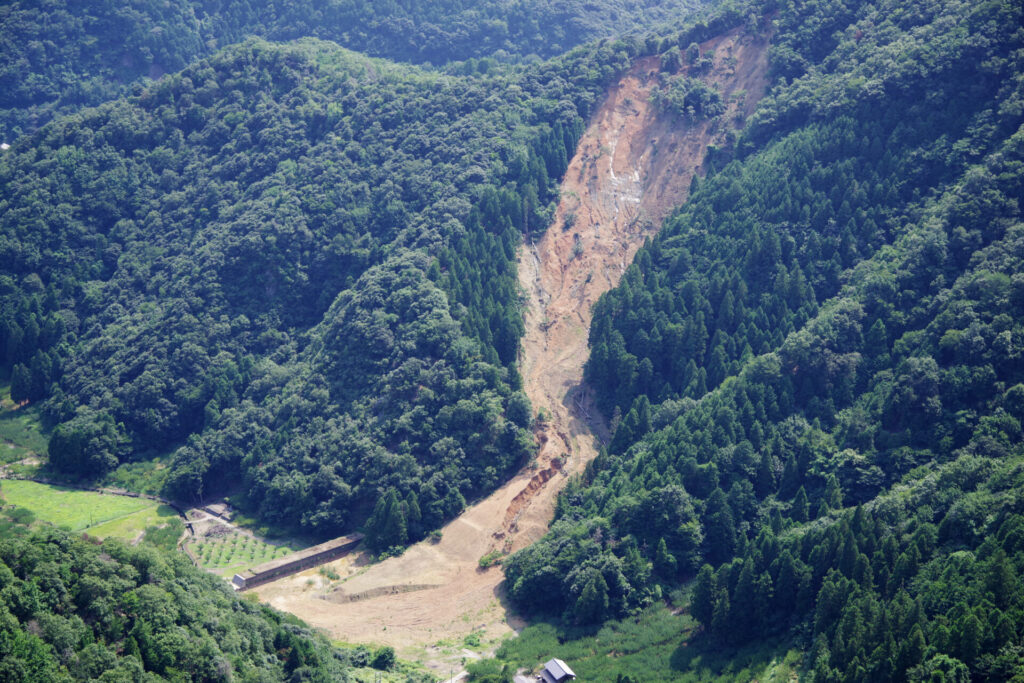
Although promising results have been achieved in various fields, including geotechnics and engineering geology, further research is necessary to fully realize the potential of machine learning and address current limitations and scepticism. One area that requires more research is scientific machine learning, which remains largely unexplored, particularly in geotechnics and engineering geology.
What is Scientific Machine Learning?
Scientific machine learning is an approach that combines traditional data-driven machine learning techniques with physics-based models to leverage the strengths of both approaches. Data-driven models are machine learning models that learn patterns and relationships directly from data, without requiring a priori knowledge of the underlying physics or mechanisms. An example of a data-driven model in geotechnics and engineering geology is the prediction of soil and rock properties by analysing large datasets of site investigation data.
On the other hand, physics-based models are mathematical models that describe the behaviour of a system based on fundamental principles and laws of physics. Physics-based models are usually formulated in the form of algebraic equations and partial differential equations, which are solved using analytical or numerical methods. Examples in geotechnics and engineering geology include conservation equations for soils/rocks as multiphase media (mass, momentum and energy) and constitutive models (e.g., Mohr-Coulomb, Hoek-Brown, etc.).
Scientific machine learning can help overcome the limitations of data-driven and physics-based models. Some of the limitations that make the use of data-driven models challenging include limited data availability and limited interpretability. On the other hand, physics-based models suffer from limitations, including assumptions/simplifications that don’t capture the behaviour of real-world systems, difficulty in model parameter estimation and computational complexity.
Combining data-driven and physics-based models in scientific machine learning can overcome their limitations, for example, through a more efficient use of data, by improving accuracy and providing interpretability to predictions. As an emerging topic, scientific machine learning is referred to using various terminologies, such as physics-informed neural networks, physics-informed machine learning and theory-guided machine learning.
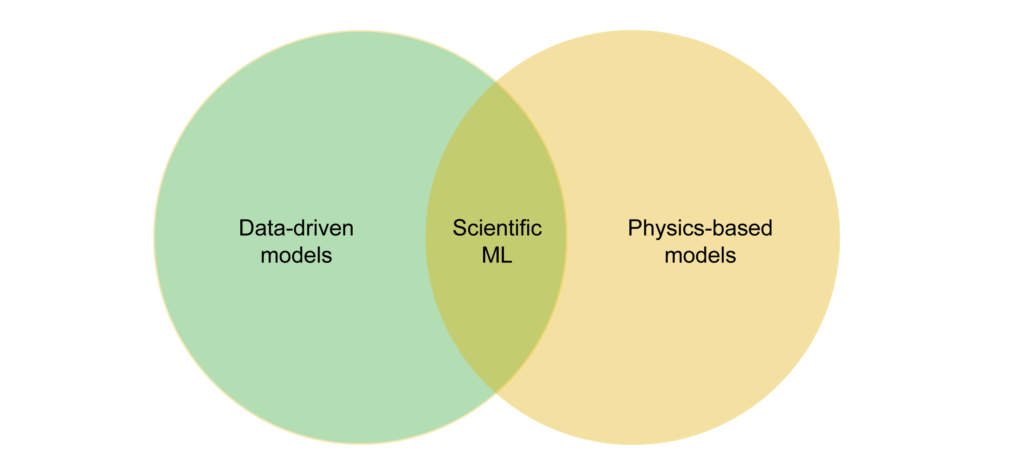
Known vs Unknown Physics in the Geosciences
Uncertainty is an inherent part of scientific inquiry and knowledge. Most scientific disciplines rely on observations, measurements, and experiments to test hypotheses and theories. However, some disciplines may encounter more uncertainty than others due to factors such as the complexity of the materials or systems they study, limitations in data availability and acquisition methods, and the difficulty of testing new hypotheses. Geotechnics and engineering geology are examples of such disciplines, which involve a significant degree of uncertainty and variability concerning the behaviour of earth materials such as soil and rock.
In some situations in rock and soil mechanics, the physics of the underlying processes are well understood and can be modelled using established theories and principles. For example, existing models based on classical and continuum mechanics can well capture the response of soils and rocks under different loading conditions.
However, in other situations, the physics of the underlying processes are not well understood, resulting in a high degree of uncertainty. For instance, natural hazards such as landslides and earthquakes can involve complex physical processes, leading to predictions characterized by a high level of uncertainty. Even in cases where the physics is considered well understood, uncertainties arise due to factors such as the variability of soil or rock properties and modelling assumptions.
Machine learning and artificial intelligence can be utilized to identify unknown patterns that may be impossible to detect using existing approaches, thereby shedding light on the uncaptured physics of the underlying processes. By coupling known physics from existing models with machine learning and AI techniques, it is possible to obtain a better description of reality.
Potential Applications
There are several application areas in geotechnics and engineering geology where neither data-driven models nor physics-based simulations alone can provide satisfactory results. Scientific machine learning could potentially be applied in these situations to benefit from the advantages of both approaches. Some potential application areas include:
Landslide/rockfall prediction: Predicting the likelihood of landslides/rockfalls is a challenging task due to several factors. These natural hazards are usually triggered by a combination of factors, such as heavy rainfall, slope geometry, soil/rock type and vegetation cover. These factors interact in complex ways that are not captured by existing physics-based models. Limited data availability is another factor that makes their prediction challenging, when using purely data-driven models. Uncertainties in existing physics-based models also lead to uncertainties in predictions. Combining data-driven and physics-based models could help in predicting landslides/rockfalls better than what is possible based on each of the approaches separately.
Digital twins of monitored geo-structures: In some situations, geo-structures (e.g., dams, tunnels, excavation supports) may need to be monitored to assess their behaviour and performance over time by installing various types of sensors. Digital twins of such geo-structures may be created to enable real-time monitoring, prediction of structural behaviour, optimization of maintenance requirements and risk assessment. These objectives of digital twins may be significantly enhanced through a combination of data-driven and physics-based models via scientific machine learning. This allows for making faster numerical predictions that would otherwise take a longer time based on traditional physics-based simulations.
Material and model parameter estimation: One of the most significant challenges in computational geomechanics is the selection of appropriate material and model parameters for geomaterials such as soils and rocks. There are several existing constitutive models for such materials that involve a number of physical material parameters and specific model parameters. While some of these parameters may be obtained from field and laboratory tests, others are more difficult to measure. In addition, parameters obtained under controlled laboratory conditions may not exactly reflect actual field conditions. A good example here is thermal conductivity, where the actual field condition is more important than individual constituent properties in a multiphase medium. To address these challenges, inverse problems that combine data-driven and physics-based models can be used to make a realistic estimation of materials and model parameters for further analysis and design applications.
Realizing the Potential
Realizing the potential of scientific machine learning in geotechnics and engineering geology requires a multidisciplinary approach that integrates several key components. High-quality data acquisition is essential, based not only on traditional laboratory and field tests but also other types of data, including sensor-based monitoring data and remote sensing data. In addition, integrating scientific machine learning with high-fidelity numerical simulations such as finite element models can enhance the accuracy and robustness of the results.
Ensuring interpretability and explainability of the results and predictions is also crucial for gaining trust and acceptance of the methods. Achieving these goals requires collaboration among personnel with diverse domain expertise and skill sets to unlock new insights and solutions to complex geotechnical and geological challenges. SINTEF is actively working on the topic and is keen on realizing the potential through research projects involving interested and relevant stakeholders.
0 comments on “The Potential of Scientific Machine Learning in Geotechnics and Engineering Geology”