Several decades ago, geophysical imaging played a vital role in revolutionising the oil and gas industry. The large-scale seismic imaging of hydrocarbon reservoirs set the standard for big data management long before the need was recognised in other sectors. The enhanced visualisation of large subsurface structures helped optimise oil and gas recovery strategies by enabling more flexible and better decision-making. Such large-scale image processing was limited to exploration and seldom thought of as applicable to production.
The flow assurance sector, which incorporates the flow lines that transport oil from wells to offshore platforms (or from offshore to onshore facilities), has not applied these technologies. In contrast to exploration activities, this sector uses meters and sensors that deliver signals in real-time, enabling rapid process control management of fast-flowing systems.
Production monitoring today and in the future
Today’s monitoring and regulation of production are based on traditional metering technologies that were originally developed several decades ago, and still used to great effect today. The main objective is to maintain stable rates of production. Some of the main challenges faced by this process are hydrate formation, scaling, sand transport and the formation of densely-packed layers (in separators). Handling most of these issues will require advances in online flow measurements in pipelines and appropriate remedies or control strategies based on an understanding of flow properties.
This article will discuss in particular how advances in measurement and sensing capabilities will promote the next innovation in the petrochemical industry. Following the shale oil revolution, the next breakthrough will be the generation of ideas about how to improve oil recovery efficiency and automation, which in turn will lead to cost savings and less expensive oil production. Advances in measuring systems will enable the acquisition of detailed information on pipeline flow properties that can be utilised to support intelligent decision-making as a means of optimising production. In the future, this will further reduce the need for human interference during production operations.
Improving measuring systems
Flow rate measurements have traditionally been performed using Venturi meters (for differential pressures) or Coriolis meters, among others, for single-phase flow. However, these methods cannot measure essential parameters such as gas, oil or water phase fractions in flow lines. Multiphase flow meters are required for such measurements. In the past, phase fraction estimation was performed using partial separators that were demanding of space and cumbersome to use. Even though state-of-the-art measuring systems are capable of carrying out high-quality flow measurements, there is still great scope for improvement.
Several types of inline multiphase meters have been developed in recent decades. Devices such as capacitance sensors and gamma densitometers are easier to use and deliver reasonable levels of accuracy. These sensors can measure gas, oil and water phase fractions along the height of a flow channel. New developments in rapid online data transfer and processing of sensor point measurements enable a better understanding of the prevailing flow pattern, as well as improvements in phase estimation.
Many research institutes and companies such as DNV GL, the Christian Michelsen Institute (CMR), FMC and SINTEF are investing in perfecting such technologies.
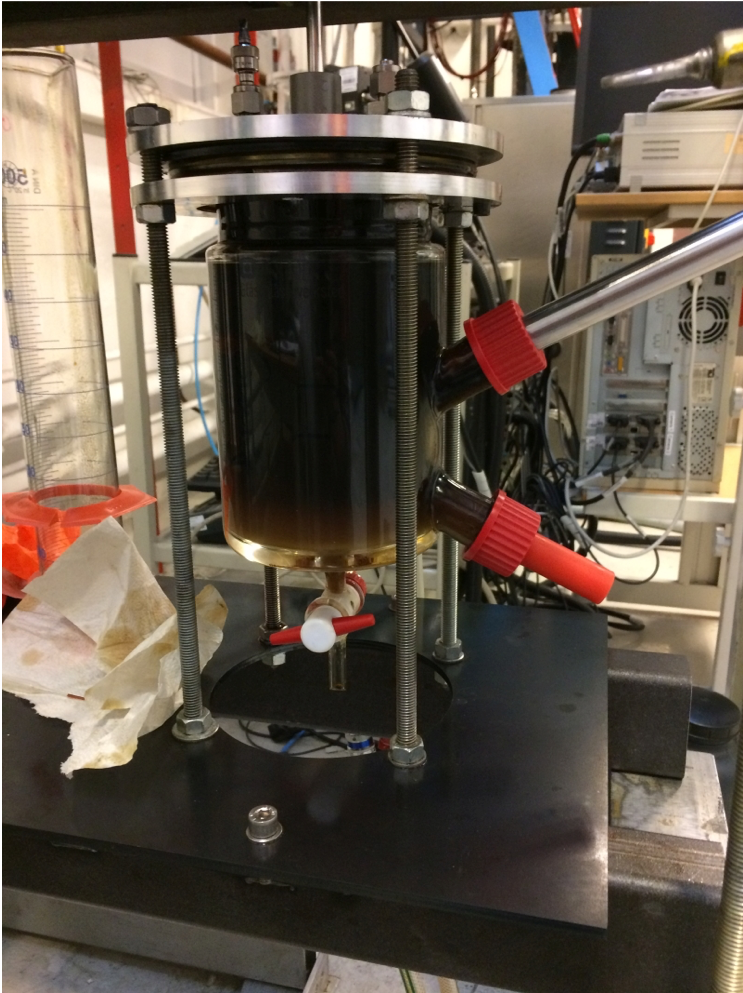
Reduceing the need for human operators
The challenge now facing the sector is how to utilise the sensor profiles acquired from various points along a flow line as a basis for intelligent decision-making and optimal operations. A more simplistic version of these technologies (called Virtual Flow Meters), which utilises pressure measurements along the flow lines, combined and neural networks, is already being used.
In the absence of rapid, real-time signal processing, it was believed that such technologies would never be viable. However, as modern processors such as GPUs become smaller and portable, these can be installed on-site and used effectively. Advancing control systems that handle large volumes of process data obtained from multiple sensors are essential for reducing the need for human operators and facilitating efficient subsea operations.
Individual flowing phases (gas, water or oil) also contain dispersed fractions of the other phases. It is impossible to measure the fraction and size of droplets/bubbles in the flowing stream using any of the available state-of-the-art approaches. In some situations, measurement accuracy in highly dispersed systems is low. The possibility of resolving this issue will open opportunities for accurate phase estimation and will enable accurate production metering. Moreover, such tools will be of great benefit to the oil/water separator process, where the formation of densely-packed layers currently hampers performance. The effective utilisation of production chemicals such as de-emulsifiers is vital for efficient operations. Droplet sensors working in real-time have the potential to revolutionise separator operation.
Bringing AI and Big Data together on a larger scale
Some new start-ups such as SOPAT have developed concepts for advanced laser and xenon probes that can monitor droplet size. Droplet images are captured by probe cameras and their dimensions measured using detection software. Rapid parallelisation methods using portable GPU’s enable on-site image processing in real-time. The statistical logging of measured droplets represents a potential first step towards a droplet sensor process in real-time. Estimation of the droplet phase fraction using distribution data is being attempted at SINTEF, and such ideas are bringing artificial intelligence and big data together on a large scale.
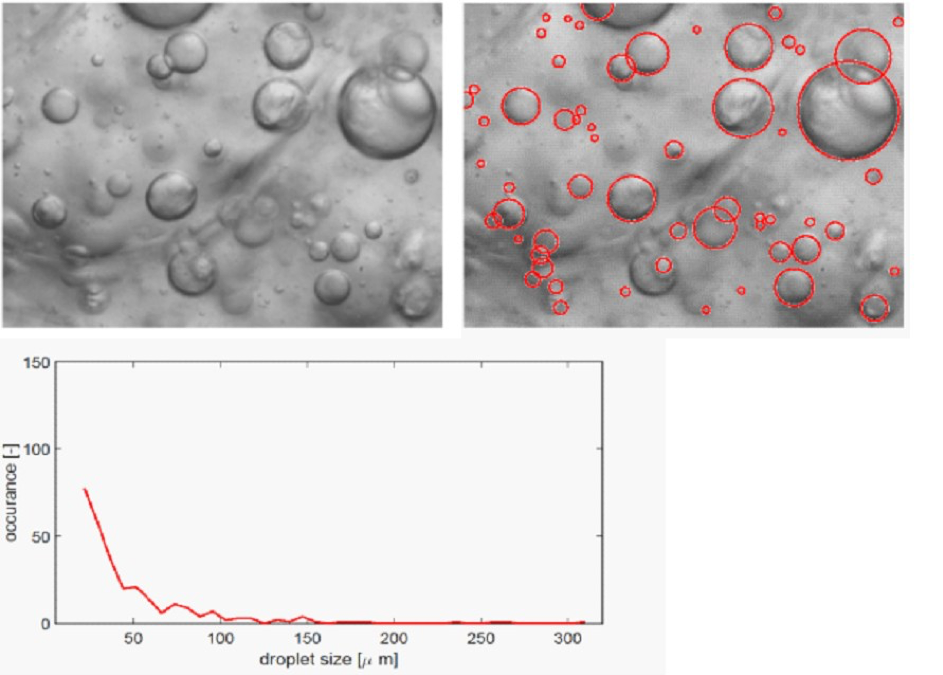
Multiple probe sensors mounted along the cross-section (height) of pipelines are able to measure the dispersion layer profile directly. However, this requires a large amount of image processing for each of the probes. Current trends in the reduction of computational speed costs will soon make this process relatively easy. Although such processes were thought of as unthinkable only a few decades ago, the large number of small GPU chips that can now be installed on-site will make such measurements possible in the near future. The future success of such monitoring devices will promote the extensive application of an advanced form of artificial intelligence, combined with big data, by the oil production sector. However, it will take years of development and proven robustness in application before the oil industry will be ready to accept such technologies.
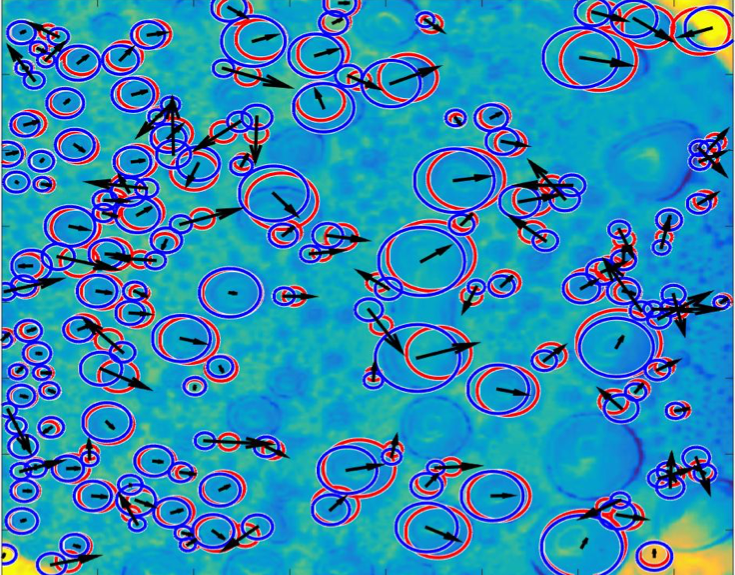
0 comments on “Artificial intelligence and big data in the petrochemical sector – probing and imaging technology: then and now”