CO-AUTHORS: STIG JOHNSEN & ASHWIN GEORGE
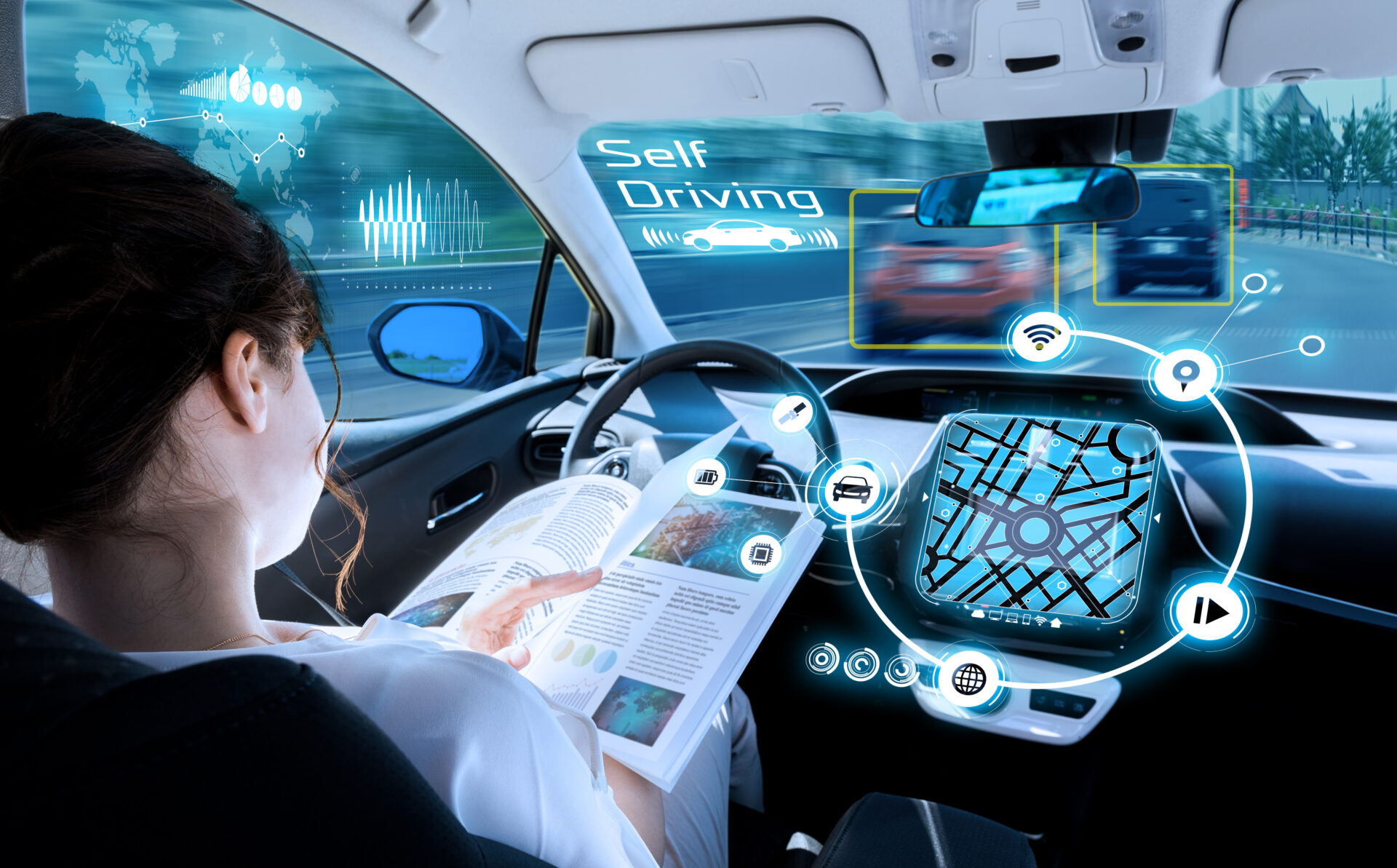
Self-driving vehicles that smoothly, quickly, and entirely autonomously drive us around – technically, we are almost there. However, before these vehicles are allowed on the road, we must ensure that they remain sufficiently safe, manageable, and responsible. How can automobile manufacturers, road authorities, and regulators work together to achieve this? In this whitepaper, we present the framework Meaningful Human Control for this purpose.
This whitepaper is a simplified and adapted version of the scientific article Designing Automated Vehicle and Traffic Systems towards Meaningful Human Control. Referencing should be made to the original paper, citing: Calvert, S.C., S. Johnsen, A. George, 2023. Designing Automated Vehicle and Traffic Systems towards Meaningful Human Control. In: Research Handbook on Meaningful Human Control of Artificial Intelligence Systems. Edward Elgar Publishing.
Introduction
The discussion on the safety of automated vehicles is not merely theoretical. Automated vehicles that fail to notice an oncoming truck, fail to recognize a tunnel, or stop in the middle of a highway – these incidents make headlines with alarming frequency. Such incidents do not help the case for self-driving cars, but the real problem is, of course, that these technical errors can cost lives.
Some argue that accidents will always happen, with or without automation. Additionally, the advantage of automated vehicles is that the technology is becoming increasingly intelligent, making automated driving safer. Given the progress made in recent years, this is not an illogical path of thought. However, by relying solely on technology as the solution, we overlook two other problems of advanced automation, namely control and accountability. With an overemphasis on technology, the complex, self-learning algorithms that drive automated vehicles can easily turn into a “black box”. It then becomes increasingly difficult for human users to understand how the system makes its decisions. In exceptional circumstances, this could lead to unexpected and unforeseen driving behaviour. If a vehicle were to cause an accident as a result, it would be difficult to establish who or what was at fault. This is unacceptable from a political and societal perspective.
Who is in charge?
The issue of safety can only be properly addressed if we consider the more fundamental underlying issue: how can humans remain in control of an automated machine?
For the current generation of automated vehicles, this issue is dealt with quite one-sidedly: the driver of an automated vehicle is always responsible. They can use the (extensive) driving assistance or automation but are expected to intervene immediately if necessary. Although this obligation may initially suffice, it is not a long-term solution. Apart from the question of whether it is reasonable to expect a “passenger” to take over the steering wheel at any given moment, the solution simply does not fit into the end picture of automated vehicles. Ultimately, we want a vehicle in which we can peacefully read the newspaper or type an email without constantly having to keep an eye on traffic.
Therefore, at Delft University of Technology, we have been looking for a broader and more robust approach. We have taken the concept of Meaningful human control as a starting point and developed it into a framework that is suitable for automated vehicles. In the following, we explain the framework and describe, with a few examples, how this approach can help automotive manufacturers, road authorities, and regulators lay the foundations for a safe, controllable, and responsible deployment of automated vehicles.
Meaningful human control
The concept of Meaningful human control was first introduced in 2015, in the context of autonomous weapons systems. Prominent scientists, entrepreneurs, and policymakers called for a ban on “offensive autonomous weapons beyond meaningful human control.” This means that automated systems must be designed and set up in such a way that humans, not computers and their algorithms, always retain control over the decisions. This ensures that humans remain morally responsible for the actions of the systems.
Tracking en tracing
This principle has since been applied to many other impactful automated systems, including autonomous vehicles. In later studies on the potential of Meaningful human control, including for the traffic and transportation sector, two conditions were described for ensuring this human supervision: tracking and tracing. Tracking entails that human reasons and intentions should always be leading. Algorithms and systems in automated vehicles should not make their own decisions without consideration of human reasons and intentions but should be designed to follow human considerations and standards. These can be fundamental, such as “do not harm people,” or more specific and subjective, such as “drive comfortably.”
The second condition, tracing, is about supervision and control: there must always be someone (a person) who directly or indirectly oversees and is responsible for the behaviour of the automated system. In the case of an autonomous vehicle, this could be the passenger, an employee in a control centre, or, in a more indirect sense, the vehicle’s programmer/designer.
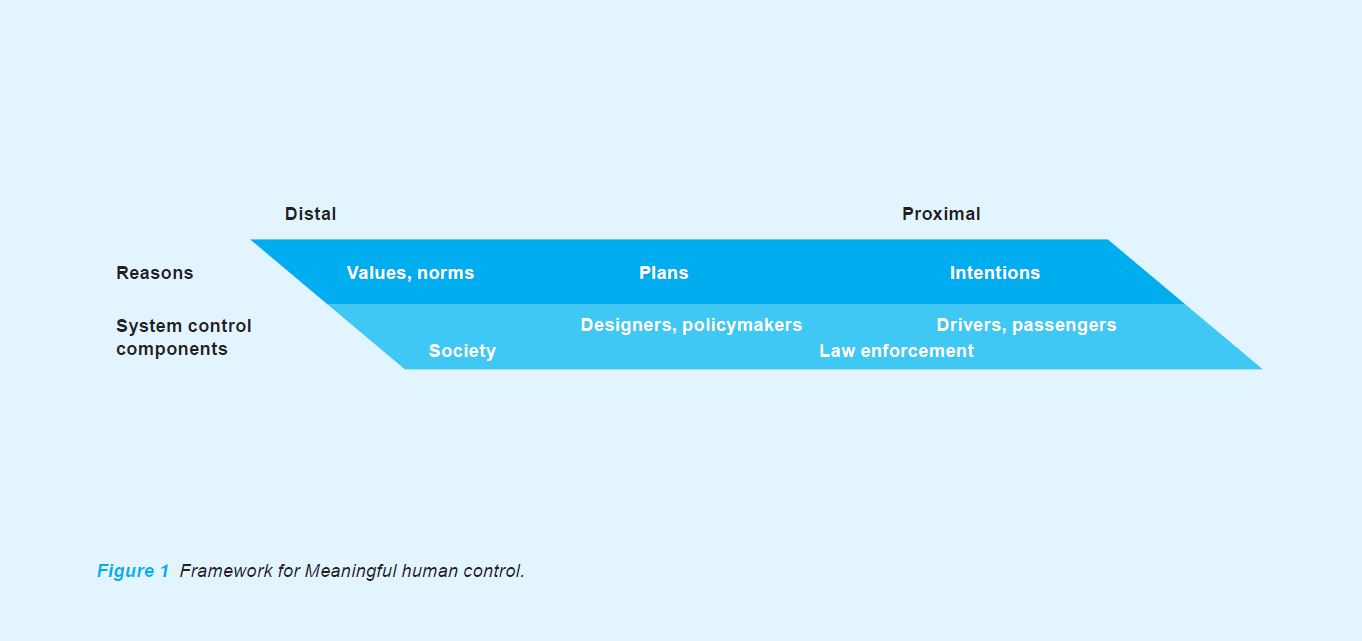
Figure 1 provides a schematic representation of the two conditions. The Reasons section concerns tracking, while the System Control Components section is where tracing takes place.1 Note that the figure distinguishes by “distance”: from more fundamental to personal reasons, and from remote oversight to control in the vehicle. We also refer to this as distal and proximal.
Integrated framework for Meaningful human control
With Figure 1, we limit ourselves to the human factor. In order to be able to consider the system of automated driving more comprehensively, we have therefore added two layers, those of the Vehicles and the Infra (Infrastructure) – see Figure 2.
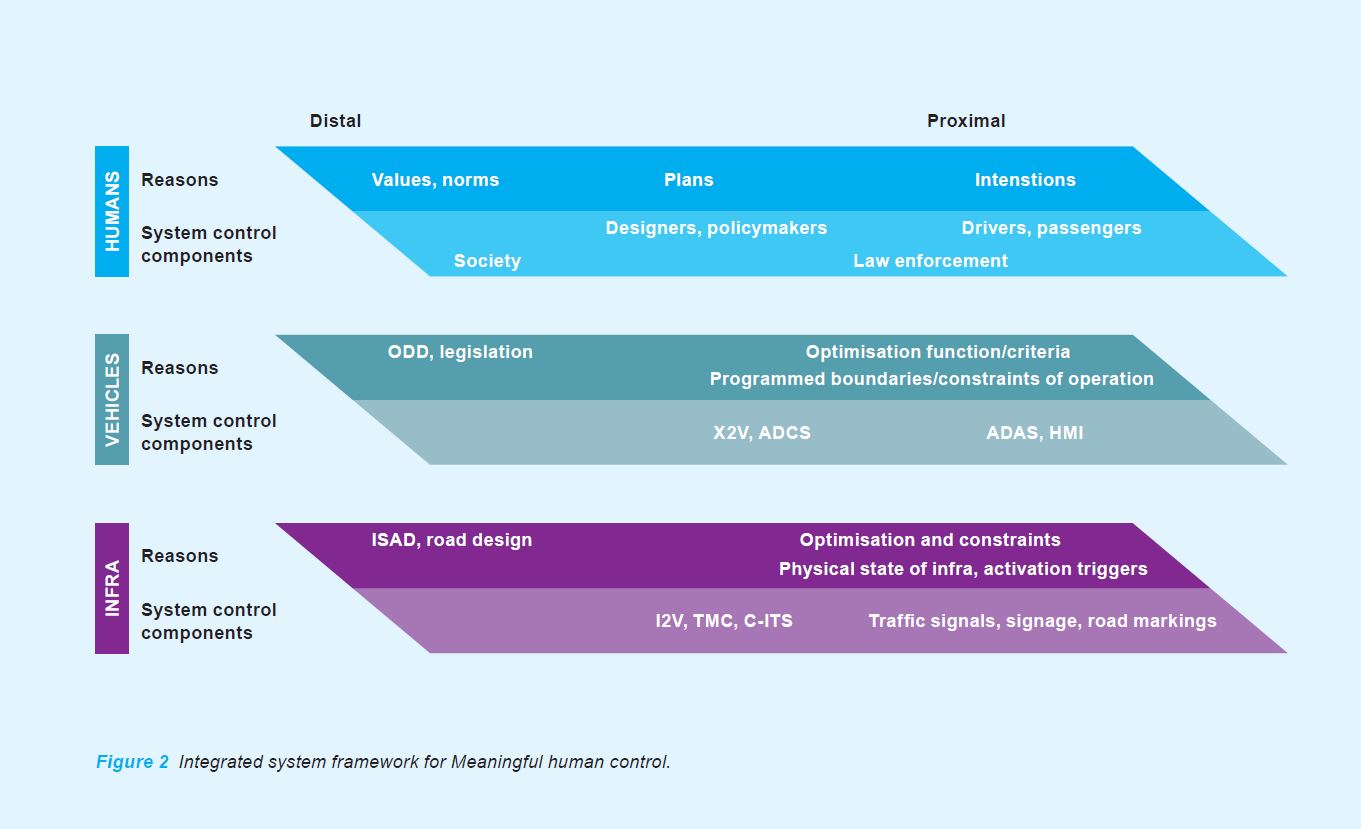
Vehicles layer
With the two additional layers, we can explicitly identify where human reasons can connect in the Vehicles and Infra layers. In the Vehicles layer, ODD[2] and legislation are listed under Reasons. These are indeed important ‘environments’ in which human reasons can (should) be integrated to ensure the proper functioning of automated vehicles. For example, if the sensors of a certain vehicle model have difficulty with low light conditions, driving in twilight could increase the likelihood of accidents and therefore collide with the human reason of ‘not causing harm to people’. This reason can be incorporated into the ODD of the relevant vehicle model by including ‘only driving in sufficient daylight’; legislation can support the reason by explicitly prohibiting the use of an automated vehicle outside its ODD.
ODD and legislation are still distal domains. Closer to the operational level, human reasons are reflected in the way the vehicle optimizes and/or restricts its control functions to interact in an environment. A vehicle can, for example, be updated to be extra cautious, with a low maximum speed and a larger distance from other vehicles.
These human reasons at the Vehicles level then guide the vehicle’s system control components. The designers and programmers of the Automated Driving Control System (ADCS), the heart of an autonomous vehicle, and Advanced Driving Assistance Systems (ADAS), for example, must adhere to the ODD, applicable legislation, and the frameworks of the control functions. This way, the reasons naturally influence the vehicle’s behaviour.
Infra layer
Something similar applies to the Infra layer, which includes both the physical and digital infrastructure. The relationships with the Humans layer are somewhat weaker, but they do exist. For example, ISAD[3] levels explicitly indicate where each ADAS system can be applied safely. And quality road markings aid an automated vehicle to only drive where it is safe to do so. Both cases relate to a human reason of, for example, ‘not causing harm to people.’
In the System Control Components section of the Infra layer, we find the traffic management and the traffic control systems, which contribute to the proper functioning of an automated vehicle in their own way. For example, the Traffic Management Centre (TMC) can guide a vehicle that is ‘stuck.’ Suppose that due to double-parked cars, an automated vehicle cannot continue to drive without crossing the central solid line. The car will not cross the line on its own initiative due to its safety settings. The TMC can then give permission to cross the line in that specific situation, with due regard for safety.
Focus on tracking and tracing
With this comprehensive, integrated framework, we can obtain a clearer picture of tracking and tracing. If we ensure that human reasons are incorporated into the Vehicles and Infra layers, the vehicles’ ADCS will naturally follow (track) the human reasons – see the green lines on the left of Figure 3. The human chain of control must ensure that the reasons are correctly implemented and are being operationally followed – the red lines on the right.
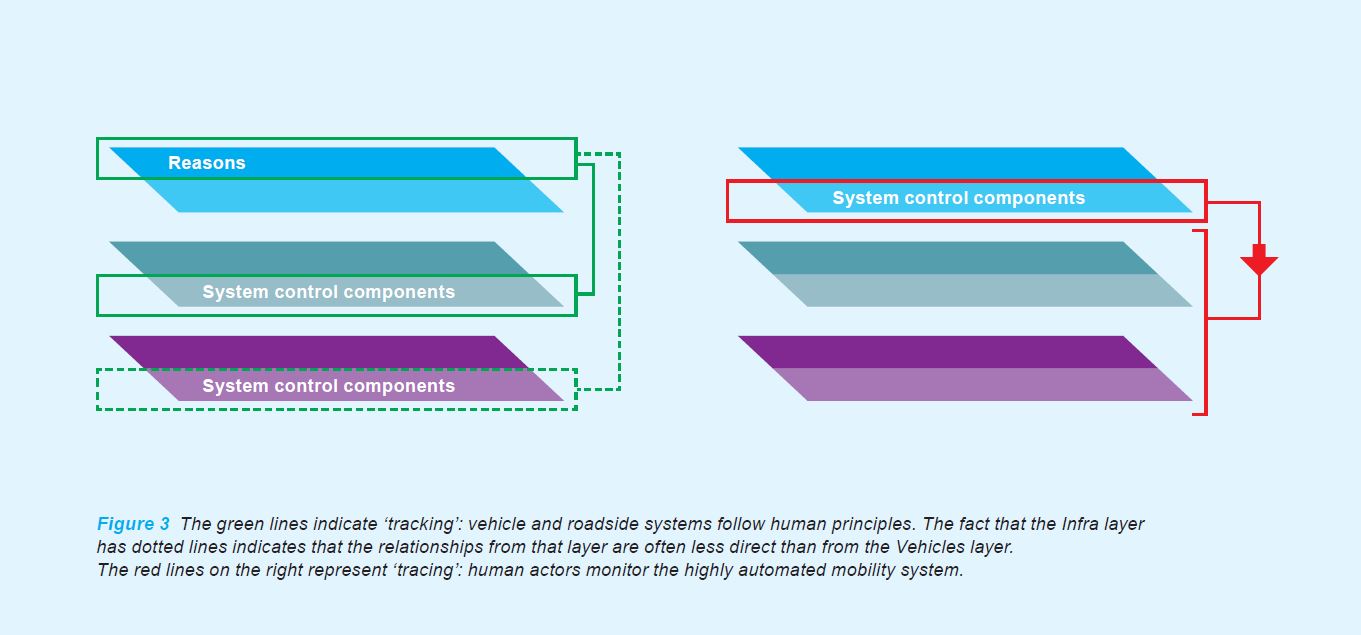
The red lines on the right represent ‘tracing’: human actors monitor the highly automated mobility system.
Process diagram for Meaningful human control
The integrated framework for Meaningful human control already allows a good understanding of how human reasons (should) be incorporated into the vehicle. However, it only offers a static view of a dynamic system. To further clarify who is responsible for what – and thereby strengthen the concept of Meaningful human control – we have developed the framework as a process diagram, Figure 4. This diagram provides a clearer view of the place and role of, for example, societal organizations and governments (regulation), and how they can influence and increase Meaningful human control.
The core of the diagram is a Connected Automated Vehicle (CAV), with two ‘control systems’, namely the ADCS and/or a human driver. Both are learning systems in principle – indicated by the blue arrows. Human drivers can improve performance through experience, while an ADCS can learn through artificial intelligence, based on its own experience or the experience of other vehicles. Additionally, it may receive software updates through wireless communication. Since this type of learning occurs internally, we call it proximal.
An automated vehicle, including the ADCS and its software, is designed and maintained by humans, referred to as vehicle designers in the figure. This category includes all parties involved in the design process of the vehicle and its components. From the perspective of the concept of Meaningful human control, these designers must ensure that human reasons and standards are properly incorporated into the ADCS. In many cases, it is wise to maintain an additional (safety) buffer. This buffer can be anything, such as asking for permission from the human driver/passenger: “Are you sure that…?”.
Of course, the human reasons that vehicle designers incorporate into the ADCS are not dreamt up out of their imagination. These reasons will largely reflect what society and regulators think and deem to be acceptable. Reasons can also enter vehicle design from government, through policies, for example. And then there are possible adjustments to reasons as a result of interaction with other vehicles (road users) and infrastructure. We just mentioned the self-learning ability, the blue arrows, which lead to optimizations. However, (near) accidents and other incidents can lead to further adaptations, such as software updates and changes in design. This may be because the vehicle designers themselves decide to do so (arrow from Interactions on the left) or because the incident has caused a stir and there is pressure from society and government (arrow from the right). We describe external interventions as distal.
Incidentally, a driver can also receive an “update”, for example through a (mandatory or voluntary) refresher course on how to improve their driving behaviour or role as a passenger in an automated vehicle. This is also a form of distal updating.
Working with Meaningful human control
Up to this point, we have introduced the two instruments for Meaningful human control: the integrated framework, Figure 2, and the process diagram, Figure 4. As mentioned, their goal is to help car manufacturers, road authorities, and policymakers achieve a safe, manageable, and responsible use of automated vehicles. However, having an instrument or even describing it is not enough – and that is why we want to briefly discuss the application of the instruments for Meaningful human control.
A substitution exercise
If we consider current practice, we see that many initiatives and efforts by car manufacturers, regulators, and other stakeholders already comply with the principles of Meaningful human control in principle. But not all, as evidenced by various incidents that have already occurred. If Meaningful human control is to really make its mark, the mind-set engraved in the principle must penetrate deep into strategies and processes setup for automated driving.
National or European governments and regulators should take the initiative here, in close consultation with car manufacturers and also representative organisations of drivers and other societal organizations. A first step could be to consider the top Humans layer of Figure 2, and consider what that means for the current state of practice and perhaps the situation in one, two, five, or more years. Which human reasons are most relevant (now or later on) in the field of traffic and transport in general and for automated vehicles in particular? Which are explicitly described in, for example, vision and policy documents? Which are not (yet)? How do they relate to each other, which are general, and which are derived? And who are the ‘control components’ on the Humans layer? Which parties are desired or even essential in this process? Who exactly are those vehicle designers, governments, and societal organizations?
The same substitution exercise can then take place for the Vehicles and Infra layers. Which ODDs and ISADs do we distinguish? What laws and regulations are in force? What are the control components at these levels? What types of ADCS are there? Which C-ITS services? And so on.
Once everything in the current and future state of play is clear, the lines of tracking and tracing can be drawn, based on Figure 3. Are all human reasons considered in the Vehicles and Infra layers? Which are not explicitly (yet)? Who has which role when it comes to supervision? Is that (human) supervision operational or more remote? And what about responsibility? Based on Figure 4, those roles and responsibilities can be filled in more sharply.
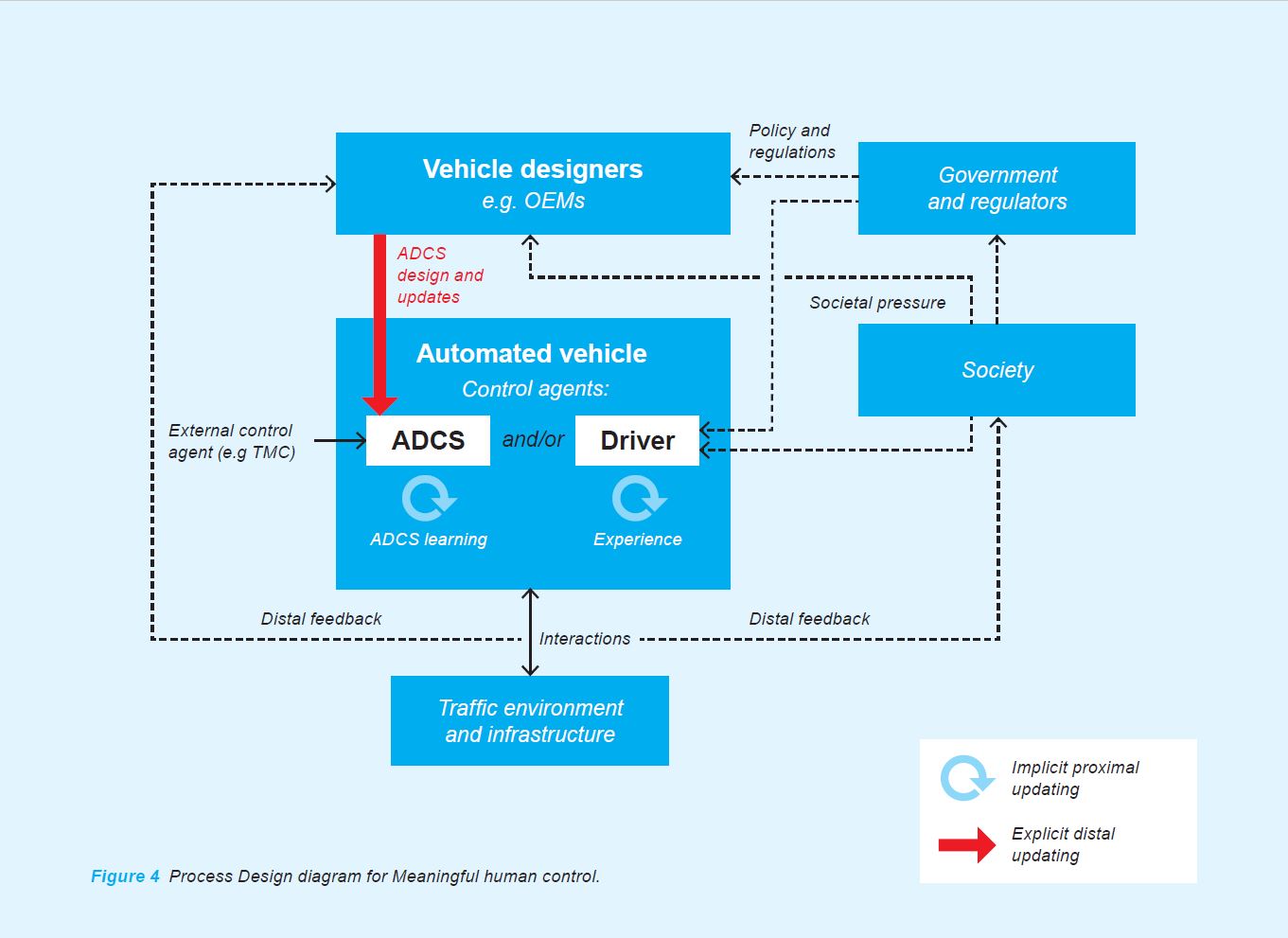
Exposing and addressing gaps
Exploring the playing field together may expose gaps, such as human reasons that are not yet explicit enough, or that are explicit but have not yet been incorporated into legislation or ODD, or perhaps even into legislation but not yet into a control system. To dig a little deeper, the parties can also go through some what-if scenarios based on the completed framework and process diagram. “If this happens or that goes wrong, who is then (morally) responsible? Are there ways to reduce the risks of the incident?”
It may not be easy, or it may even be impossible to detect and solve all these gaps immediately in this theoretical way. But exploring them systematically with the help of a framework is an essential first step. After such a joint exploration, there may also be sufficient support for regulations on Meaningful human control in work processes. For example, car manufacturers or road authorities could be obliged to include Meaningful human control in their design and management processes and to report on this in an action plan. If an incident does occur, the joint exploration and any action plans can be used to retroactively determine what went wrong and how Meaningful human control can be further integrated into the processes.
In conclusion
In this contribution, we discussed how we can keep automated vehicles safe, manageable, and responsible. Focusing only on technology and innovation is not sufficient. Primarily, we need to ensure that humans remain in control of the vehicles, even if not operationally. To achieve this goal, the concept of Meaningful human control is highly appropriate. The notion is that automated systems should be set up and designed in such a way that humans, not computers and their algorithms, always retain control over moral decisions. This way, humans remain morally responsible for the actions of the systems.
In this research, we have developed an Integrated Framework and a Process Diagram for Meaningful human control. These two tools help to visualize the lines of tracking and tracing: how are human reasons embedded in vehicle and infrastructure systems, and how is supervision regulated? Governments, car manufacturers, regulators and other stakeholders can use these tools to make their processes, roles, and responsibilities transparent and, if necessary, sharpen them.
By systematically including the human factors in shaping a mobility system with automated vehicles, we can prevent accidents and/or undesirable effects as much as possible. This way, humans will remain sufficiently in control of these increasingly smarter, but sometimes unpredictable vehicles.
References
- The figure is based on the Fundamental diagram of meaningful human control proximity of Santoni de Sio and Mecacci (2021). ‘Designers, policymakers’ have been added.
- The ODD, Operational Design Domain, of an automated vehicle describes under which conditions it can operate autonomously (where and under which conditions it may drive.)
- ISAD stands for Infrastructure Support for Automated Driving. Different levels are distinguished, indicating to what extent the road is ready for driving assistance and automated vehicle systems.
About the authors
Simeon C. Calvert, PhD, is an assistant professor at the Transport & Planning department of Delft University of Technology. He is also co-director of the Delft Data Analytics and Traffic Simulation Lab (DiTTlab) and the CiTy-AI-lab. His research is focussed on the impact of technology on road traffic. E-mail: s.c.calvert@tudelft.nl
Stig O. Johnsen, PhD, is a Senior Researcher at SINTEF (Safety and Reliability Group) and lecturer at NTNU and NORD. He is responsible for the Human Factors network (HFC) in Norway and is chair of Accident and Incident Investigation at ESRA. His research is focused on meaningful human control of autonomy in transport and oil and gas industry.
Ashwin George, MSc, is a PhD candidate at the Human-Robot Interaction Group in the department of Cognitive Robotics at the TU Delft and is part of the HERALD Lab. He explores how the introduction of technology in traffic can lead to behavioural adaptations and ethical challenges.
Comments
No comments yet. Be the first to comment!