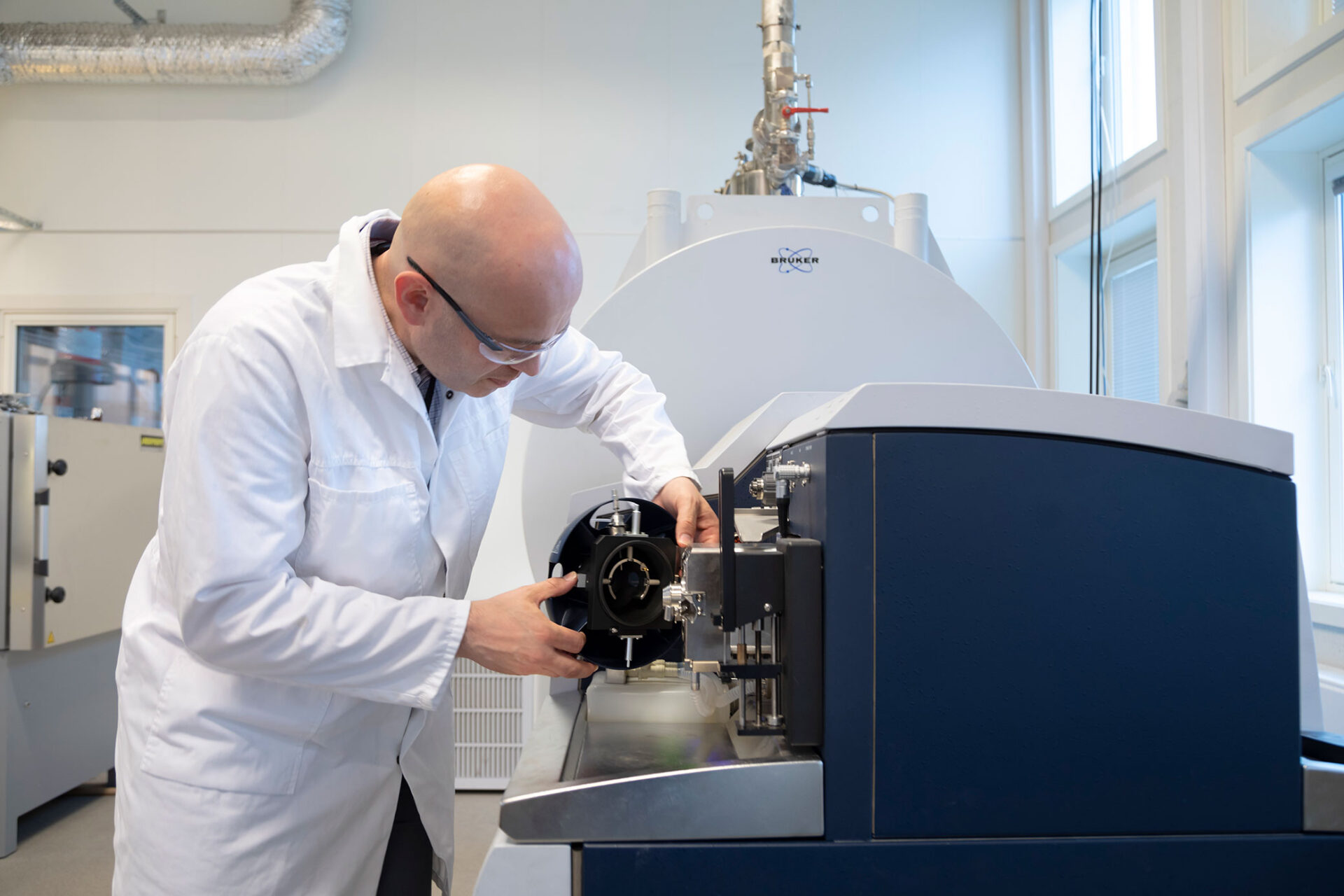
In the competence building project KPN New Hydrate Management, SINTEF and NMBU combine new experimental methods to extract specific components from crude oil with machine learning to analyse and identify these components.
Gas hydrates are ice-like structures formed under high pressure and low temperature. Water molecules are organised in lattice structures where gas molecules are trapped. These lattice structures can grow to become particles. The surface of hydrate particles is initially water wet, and two hydrate particles can stick together by water bridging. This can eventually result in big lumps of hydrates, or the hydrates can attach to a pipe wall and grow until the pipe is blocked.
Hydrate management – energy demanding and dangerous
Gas hydrates can plug pipelines and process equipment and is one of the major flow assurance challenges during oil and gas production, especially subsea in cold waters. The traditional methods for handling gas hydrates contribute to a significant part of the emissions from the petroleum sector, mainly by the use of large amounts of heat and chemicals. A recent case study concluded that CO2 emissions from oil production can be reduced by 20-30% if produced entirely without using traditional hydrate management methods*. How can this be achieved? It has been known for decades that some crude oils are non-plugging due to naturally occurring components in the crude oil, and these oils can be transported safely even with hydrates present.
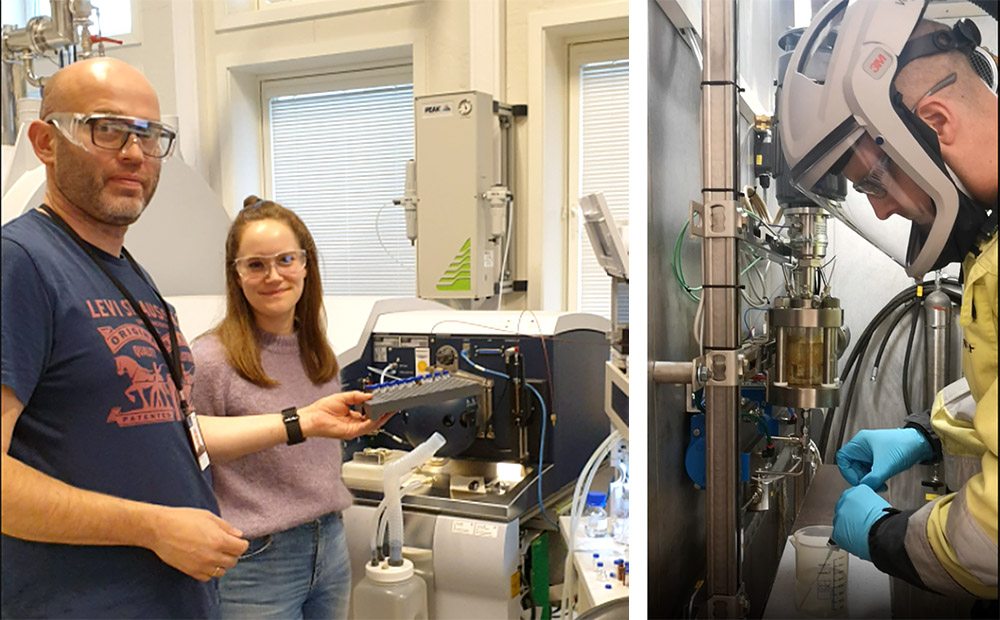
What are natural hydrate inhibitors?
Which components are they? We do not know yet, but previous studies performed by SINTEF, Equinor, TotalEnergies and others indicate that specific organic acids and other complex molecules like asphaltenes can contribute to the natural hydrate inhibiting properties. These components have not yet been identified, mainly because of the general chemical complexity of crude oils and the presumed low concentrations of these naturally occuring hydrate-active components.
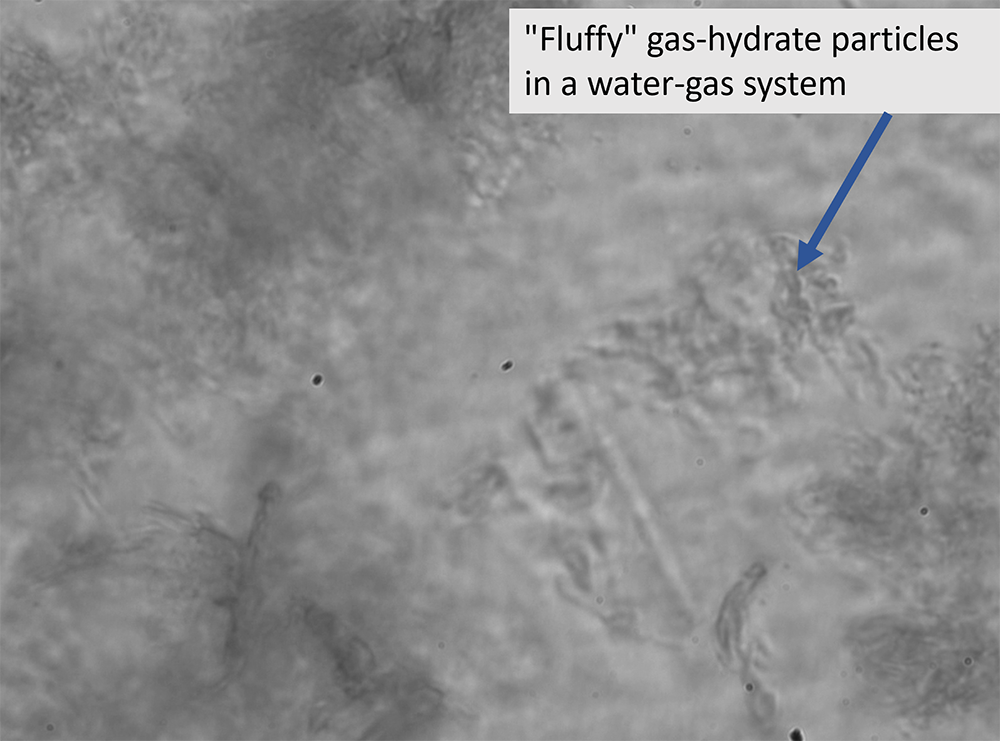
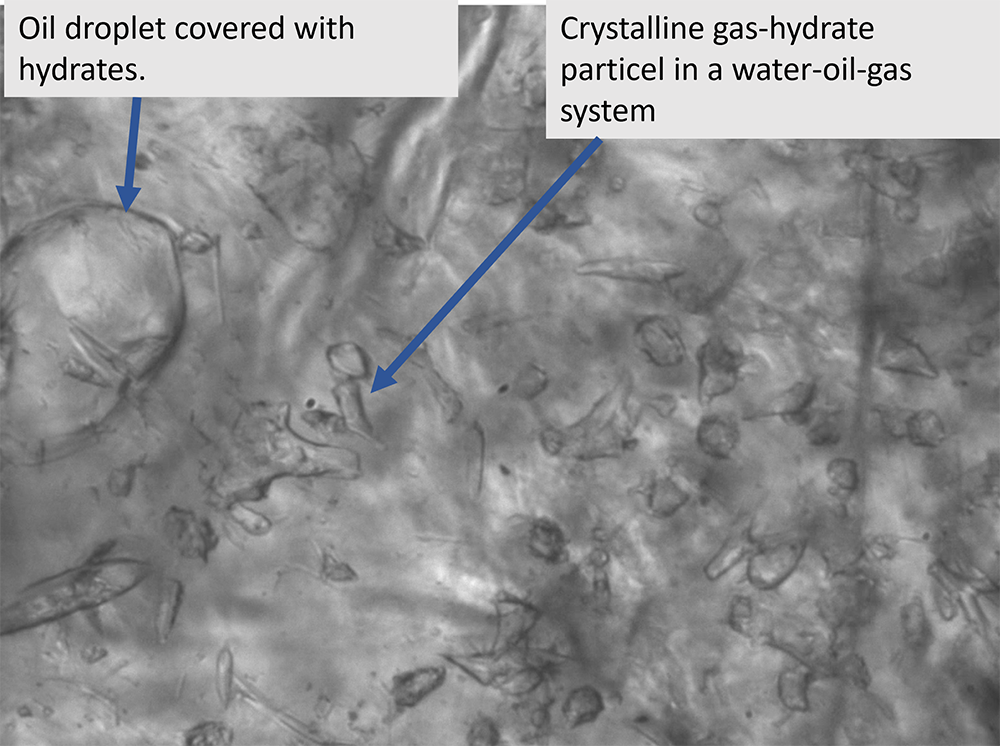
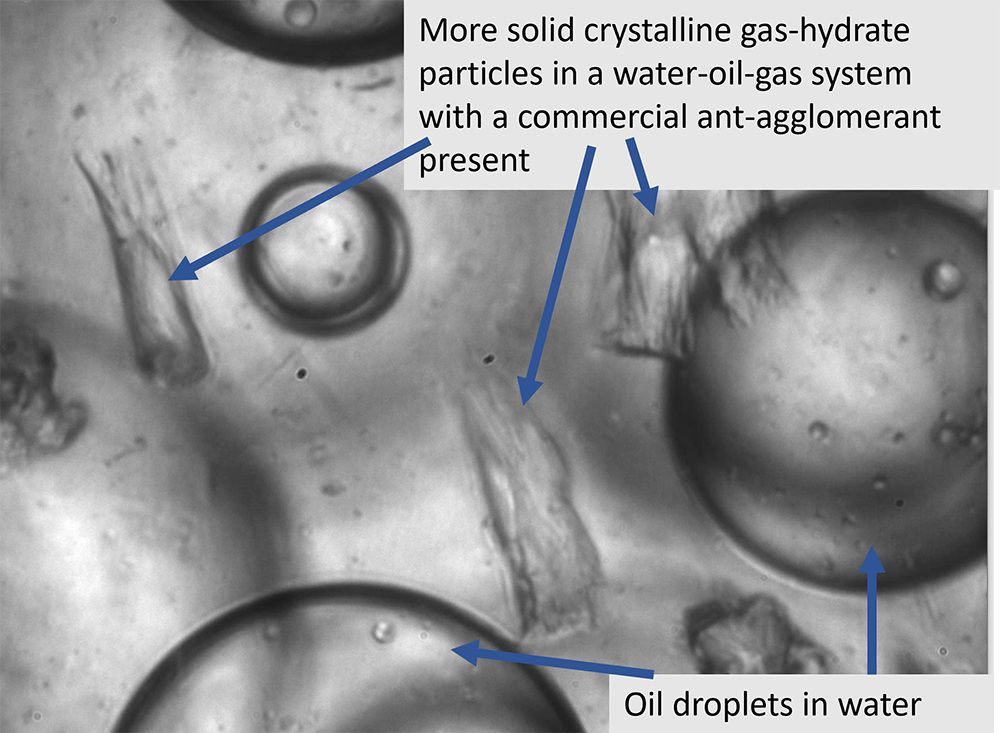
Machine learning to find “the needle in the haystack”
In the competence building project “New Hydrate Management” SINTEF and NMBU use machine learning to analyse large and complex data sets. The data are generated by a high-resolution mass spectrometer (FT-ICR MS), developed to precisely distinguish between components at molecular level. The high resolution of FT-ICR MS compared to other mass spectroscopy methods can be illustrated as follows: While the latter sees a forest from a bird’s eye view, FT-ICR MS can study the forest floor and see every shrub, leaf of grass, moss, and mushroom. In the project KPN New Hydrate Management, FT-ICR MS data from many different crude oils are analysed and compared with knowledge about these oils innate hydrate properties. These large and detailed data sets require advanced multivariate data analysis and machine learning to find “the needle in the haystack”, that is the hydrate-active components responsible for non-plugging gas hydrates.
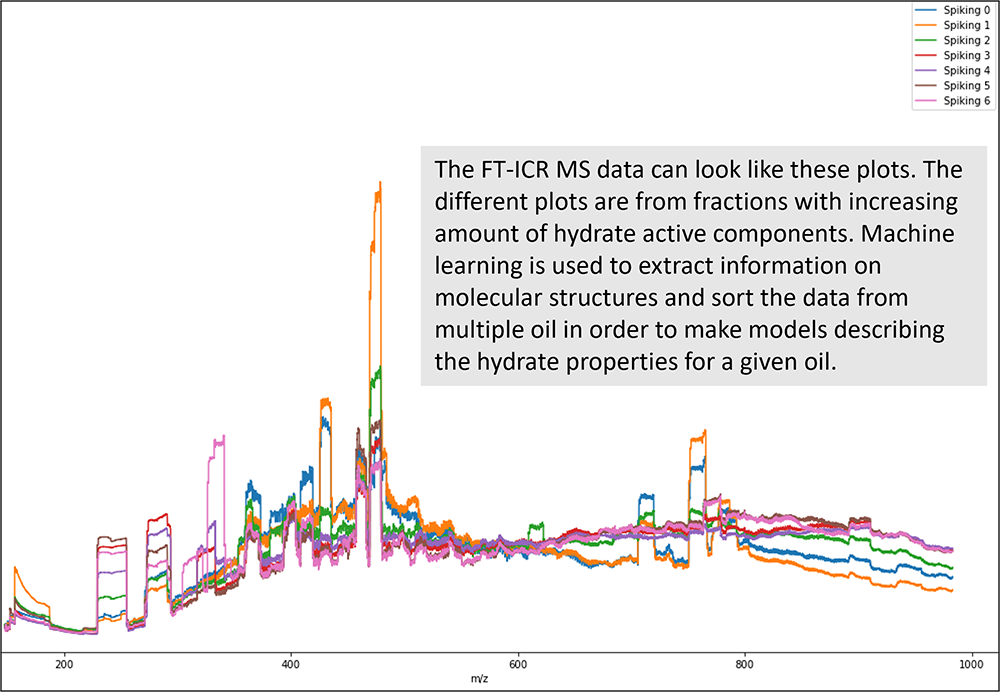
Environmental benefits through knowledge
The results and knowledge from the project will be used to develop methods that can significantly reduce the environmental impact from oil production. This can be achieved by safely producing oil within the hydrate stability zone without using traditional hydrate management strategies, thereby reducing energy and chemical consumption.
Results through research
Several crude oils with different hydrate properties have been tested at SINTEFs Multiphase Flow Laboratory. Samples have been extracted and analysed at SINTEFs lab at NTNU. The first analyses show that we have been able to concentrate the hydrate-active components. We have also been able to improve the hydrate properties of a crude oil by adding the concentrated hydrate-active components. New methods have been developed within multivariate data analysis to find correlations between oil composition and hydrate properties. Through further work planned in the project we have a good hope of getting closer to finding the “needle in the haystack”, i.e. the hydrate-active components that make crude oils non-plugging. If we succeed it will be an important contribution to more sustainable energy production. In addition, the methods developed within machine learning and data analysis will be valuable also outside the petroleum industry.
* Technical and environmental evaluation of a hydrate cold flow technique to produce an oil reservoir using a ling tie-back and comparison against traditional development concepts, the 41st International Conference on Ocean, Offshore and Arctic Engineering (OMAE 2022) in Hamburg, Germany in June 2022
Publications:
- Successive accumulation of naturally occurring hydrate active components and the effect on the wetting properties (OFCS 2021)
- Machine learning as a basis for better understanding of flow assurance through FT-ICR-MS analysis of gas hydrates (OFCS 2021)
- Towards a machine learning based produced for interpretation of mass spectra for better understanding of hydrate phenomena in oil systems (SSC17 2021)
- Identifying Components Related to Hydrate Formation by Machine Learning-based Variable Selection(OFCS 2022)
- A new high pressure method for successive accumulation of hydrate active components (ECGH2022, June 13-17, accepted)
- Utilization of machine learning on FT-ICR MS spectra for improved understanding and prediction of the properties of hydrate-active components (ECGH2022, June 13-17, accepted)
Project support and partners
KPN New Hydrate Management is supported by the Research Council of Norway (project number 294636). NMBU, OMV, Wintershall DEA, Equinor and TotalEnergies are partners.
Comments
Congratulations on your work. Please don’t forget that hydrates are freezing together with waxes, a combined depósiton problem. Good luck