In February, together with financial technology (“FinTech”) startup NorQuant of Oslo, we arranged a half-day seminar “Machine Learning in Finance” open to anyone interested.
Promotion of the event consisted only of sharing of invitations on LinkedIn. To our amazement, around 250 attendees turned up. Even more amazing, these were representatives of heavy-weights in Norwegian finance; large banks, insurance companies, mutual funds, the central bank, and NBIM.
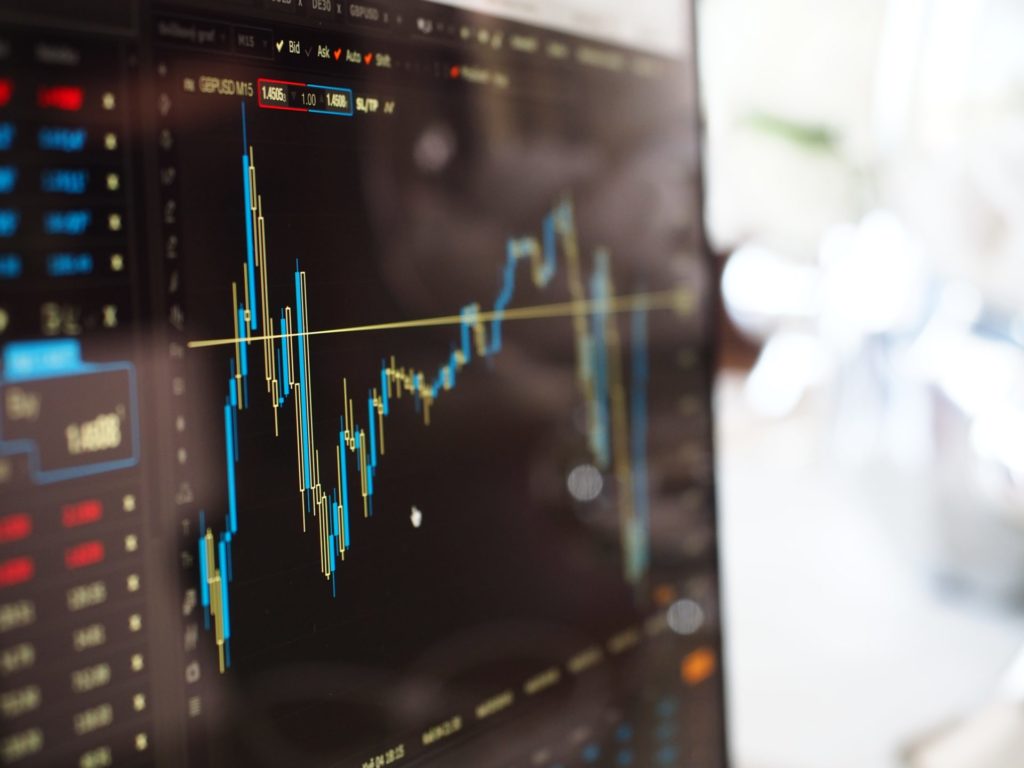
This tells us one thing: There is a surge of interest out there, and SINTEF is equipped to bring something unique to the market. Most of our experience is from working with other sectors than the financial industry, but why not turn this to an advantage?
We are not mired in predispositions. We know computer science, mathematics, and machine learning. We know people. We use financial services. And, we work with an aim of technology for a better society!
Modelling, statistics, machine learning in Finance
The Financial Services industry is one of the industries most impacted by the recent surge in digital technologies and digital transformation.
Many financial institutions start to see themselves more like technology companies.
At SINTEF we see a clear potential for using our expertise in fields like modelling, statistics, machine learning and optimization to be a research and development partner for such institutions.
As an example we bring relevant experience learned from the oil industry. Offshore drilling of wells for oil production is a high cost activity, and obviously one where safety is highly critical. So, the incentive to work efficiently needs to be balanced with keeping within safe operating conditions. Monitoring what is happening in the well while drilling is not trivial though, as the conditions are harsh, and the action potentially happens several thousand meters below the sea-floor. As a result of this, decision mechanisms are complex, and often depending on data which is not directly measurable but where mathematical models produce a synthetic data based on what can be measured.
SINTEF sees great potential in combining models derived from physical understanding of the system with purely data driven machine learning models.
A similar approach can be pursued towards modelling market behaviour with the purpose of identifying and potentially automatically executing trading strategies. Well known market dynamics can be represented as classical models and be enhanced by use of data driven models based on alternative data sources where influence on market behaviour may be less understood and difficult to interpret by humans.
Additionally, the abovementioned approach to drilling of wells puts requirements to system explainability. In order to comply to safety regulations, operators need to understand the behaviour of control and safety systems at some level. These challenges can be transferred to financial services, where e.g. decisions on whether a client is creditworthy should be explainable to ensure fair treatment of individuals
In a press release from December 2018, Gartner states that “27 Percent of Finance Departments Expect to Deploy Artificial Intelligence by 2020”, according to a survey they have conducted. Based on extensive experience across industries, SINTEF Digital can play a role in this transformation.
Machine Learning in Finance may be a good tool to solve many challenges
There are many challenges to handle:
- Price discovery,
- portfolio management,
- risk management,
- anomaly detection,
- credit scoring,
- security,
- pattern recognition,
- bet sizing,
- asset allocation.
Machine learning may be a good tool for many of these. We can help building models to solve these challenges. Furthermore, we can help with research into how these models will work. In 2020 and beyond, a working ML-model will not be enough. Both designers, users and authorities will require knowledge on what is termed “explainable AI”. Maybe this will be SINTEF’s greatest contribution to Machine learning in Finance in the future?
0 comments on “Machine Learning in Finance”